Historically, the stormwater sector has struggled to communicate to communities the inherent uncertainty involved in predicting tomorrow’s extreme storms. Ask the average person to define a “100-year storm” and they most likely will describe an event expected to occur only once every century. In reality, a 100-year storm is an event with a 1% chance of occurring in a given year. And that percentage is likely to fluctuate over time as impervious space dwindles with development and major storms become more frequent with climate change. Likewise, the average person might conclude from a flood map that if their property sits outside an area expected to flood during an intense storm, they will not experience flooding even under extreme circumstances.
A pair of researchers from the University of Georgia (UGA; Athens) are borrowing concepts from the world of statistics to create more informative, transparent, and accessible flood maps. The team recently published an open-access study describing their work in the journal Water.
“Conventional flood hazard mapping tends to draw a single line on a map showing the flood zone, which is often interpreted by the public and politicians as, ‘You’re not going to get flooded if you’re outside the line,’” said study co-author and UGA engineering professor Brian Bledsoe in a release. “In reality, that line can be very uncertain and fuzzy, and a large proportion of flood damages occur outside of it.”
Introducing the SUB Method
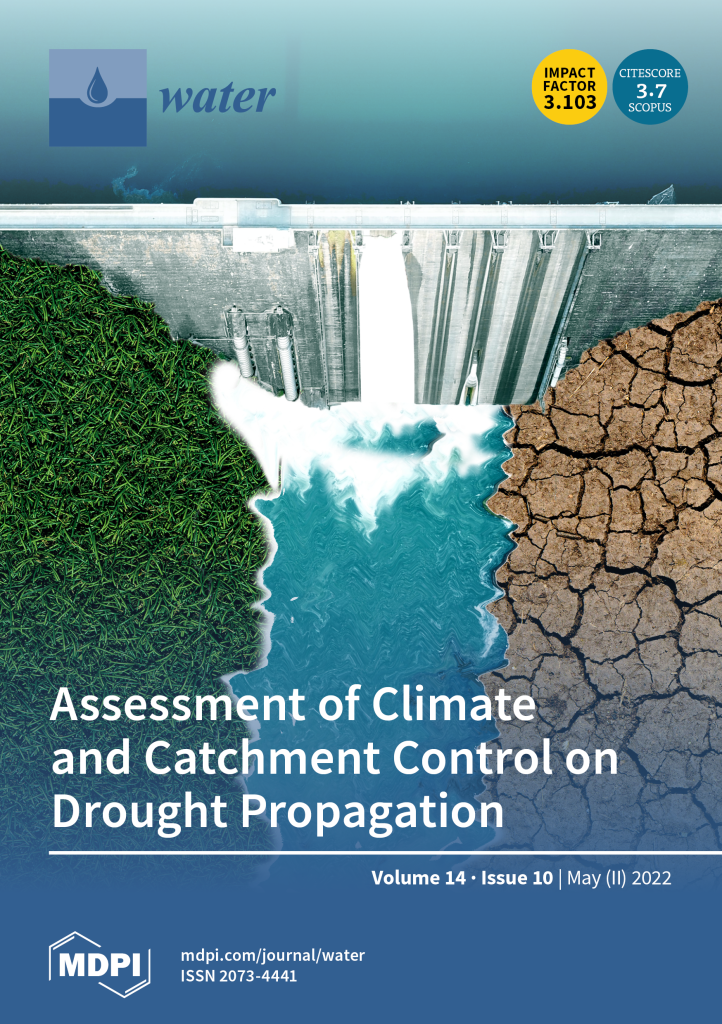
Statisticians often perform a technique called Monte Carlo simulations to incorporate unknown or random variables into predictions of whether a particular event will occur. In the case of predicting flooding, however, the sheer number of uncertain variables —the speed, location, volume, and timing of rainfall as well as how it interacts with such geographic factors as waterbodies, cityscapes, and slopes — make Monte Carlo simulations particularly complex.
For that reason, devising flood maps that accurately account for uncertainty typically is feasible only for large, well-resourced municipalities able to invest in advanced computer models, study authors describe. Even then, creating these flood maps typically requires running thousands of Monte Carlo simulations to cover a full floodplain. Consequently, many Monte Carlo-based flood maps provide information for only a few specific points of interest rather than a complete region.
Bledsoe, along with recent UGA engineering alumnus Tim Stephens, propose a far less complex alternative to Monte Carlo simulations as a foundation for creating risk-based flood maps. They call this method simplified uncertainty bounding (SUB).
“We use advanced tools to quantify the uncertainty around flood lines and describe the full range of locations where flooding is likely to occur,” Bledsoe said.
Similar to Monte Carlo simulations, the SUB method outputs a percentage conveying the likelihood that a specific point outside a known flood-prone site will experience flooding under different storm scenarios. But unlike Monte Carlo simulations, which require immense amounts of data to make accurate predictions, the SUB method uses only a few datapoints to create a rough picture of the floodplain. The method infers missing data based on patterns. While the SUB method’s predictions often lack the detail of those developed using Monte Carlo simulations, they can be formulated at a fraction of the time and cost and are typically still similar enough to provide useful information.
Insights at a Fraction of the Cost
The researchers put the SUB method to the test by creating flood maps using both Monte Carlo simulations and their new approach in two contrasting urban watersheds: one surrounding Proctor Creek in Atlanta and another encircling Bronx Wash in Tucson, Arizona.
In each study area, the team performed 1,000 Monte Carlo simulations and only two SUB simulations to identify areas that were at least 90% likely to experience flooding during a localized 50-year and 100-year storm. Both strategies drew on such known factors as floodplain topography and elevation, approximate runoff discharge rates for each simulated storm, and existing built or natural flood control infrastructure.
In general, the study found that the areas identified as flood-prone by the SUB-developed maps were only about 10% different than those of the Monte Carlo maps. However, the exercise revealed several factors that affected the SUB method’s usefulness for predicting flood hazards.
Perhaps most significantly, the method did not estimate the flood-mitigation effectiveness of existing infrastructure nearly as comprehensively as Monte Carlo simulations. The SUB method either over- or under-estimated existing infrastructure effects based on other, site-specific factors. The new method also tended to overestimate flood risks in the case of more intense storms and underestimate flood risks in the case of less intense storms compared to the Monte Carlo method, the study describes.
The research team acknowledges that the SUB method may not be an appropriate fit for every floodplain manager. However, they emphasize that it is far more accessible and provides comparable outputs to those developed using far more complex approaches.
As Bledsoe and Stephens continue to study low-cost ways to account for uncertainty in flood prediction, they also are working alongside such partners as the U.S. Army Corps of Engineers, the U.S. Federal Emergency Management Agency, and social scientists to devise better strategies to communicate their work to homeowners and the public, Bledsoe said.
“For example, we’ve found that saying things like, ‘You can be a certain percentage confident that your home will not be flooded in the next 20 years’ is much easier to grasp than abstract statements about the 100-year flood or 1% annual exceedance probability,” Bledsoe said.
Top image courtesy of Markus Distelrath/Pixabay
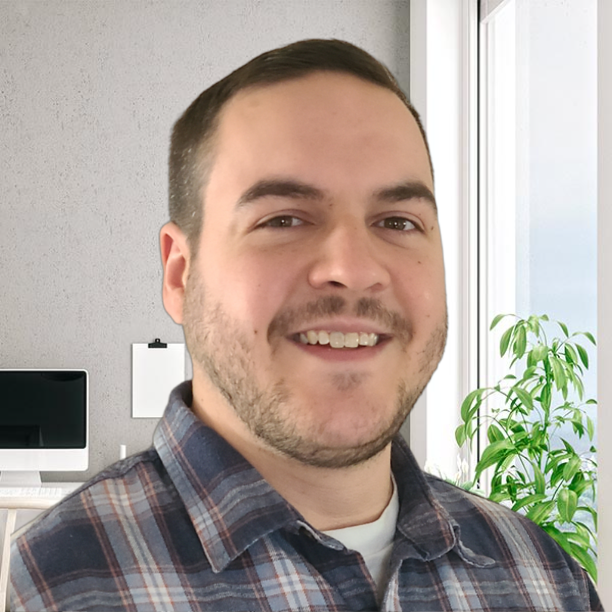
ABOUT THE AUTHOR
Justin Jacques is editor of Stormwater Report and a staff member of the Water Environment Federation (WEF). In addition to writing for WEF’s online publications, he also contributes to Water Environment & Technology magazine. Contact him at jjacques@wef.org.