During an extreme storm event, useful information about the safety and accessibility of specific roadways makes all the difference for decisions including whether and how to evacuate, the best ways to secure emergency supplies, and how to prioritize rescue efforts. The stakes of whether this information is accurate and up to date can be life or death: Driving vehicles through flooded roads is the most common cause of flood-related fatalities in the U.S., and as little as 15 cm (6 in) of water can make most vehicles uncontrollable.
Many tools — from traffic cameras and water-level sensors to satellites and social media posts — can provide this information, but individually, each has its weaknesses. Traffic cameras, for example, often focus only on the busiest roadways, neglecting other parts of the network. Water-level sensors provide high-resolution data but are excessively expensive to deploy on a municipal scale. Satellites and drones can canvass large areas, but typically only for specific points in time rather than providing continuous coverage, and they frequently face visibility issues during heavy storms. Social media posts are subject to bias and noise, requiring time-intensive manual interpretation to become useful.
At Rice University (Houston), researchers are exploring the use of artificial intelligence (AI) to combine an array of different information sources into a single, real-time tool. The resulting framework could one day enable locals and first responders to access up-to-the-minute information on the safety of virtually any urban roadway during a major storm, according to Jamie Padgett, Rice University structural engineer and co-author of a recent study about the effort published in Reliability Engineering & System Safety.
“This study offers communities a pathway to equitably sense and respond to urban stressors such as flooding using existing data sources,” Padgett said in a release. “Here, we focus on flood impacts on transportation infrastructure and focus on understanding how other data sources can complement information from flood models, especially with respect to impact on roadways and safe mobility.”
Fusing Several Sources of Data
To help guide the development of their AI framework, the researchers began by conducting in-depth interviews with 24 stakeholders — technologists, emergency response personnel, academics, and others — while also gleaning insights from previously created AI-based data fusion platforms. They decided on several sources of data from which their tool should draw. These included
- physical sensors, such as traffic cameras, traffic speed, and roadway water-level gauges;
- social sensors, such as citizen information reported to municipal agencies and social media posts;
- physics-based models detailing how stormwater is most likely to move throughout an urban watershed; and
- “human-in-the-loop” input, in which humans participate to some degree in the training and interpretation procedures of the AI model’s machine learning processes.
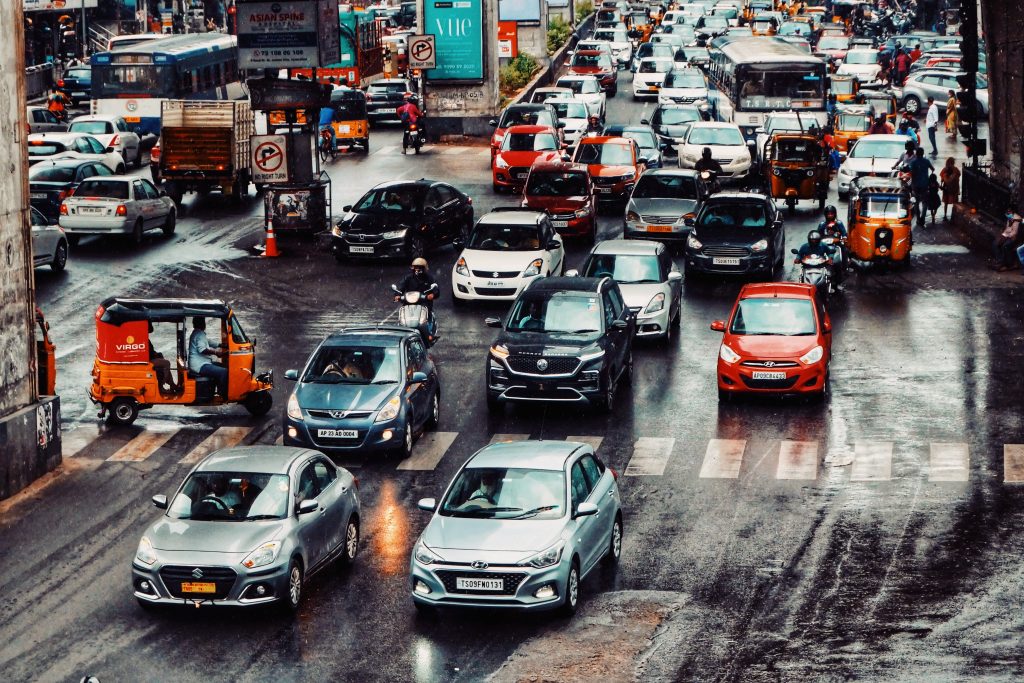
As a growing collection of human-assisted AI models are demonstrating in various sectors, including human-in-the-loop parameters is a way to provide quality control and improve prediction confidence in a way that does not significantly hamper processing time — which is particularly important in systems designed to advise safety decisions.
“We do not want a system that is fully automated and without any human control,” said co-author Pranavesh Panakkal, a Rice University postdoctoral researcher in civil and environmental engineering. “The model may make a wrong prediction, which could endanger community members who decide to risk travel based on this prediction. So, we designed safeguards based on responsible AI usage. This need for responsible AI in such tools is still an open area for further work, and we hope to dig deeper as we test our methods in the future.”
With sufficient data and training, the resulting framework can make confident, up-to-the-minute predictions about whether a particular roadway is likely to be flooded at any given moment. Insights from the tool populate a freely accessible, web-based dashboard. This brings the potential for cities and their residents to maximize situational awareness without significant investment in new sensors, researchers say.
Revisiting Roads During Hurricane Harvey
In Houston, timely data availability proved a pain point during Hurricane Harvey in 2017. Lacking real-time information about roadway conditions, many emergency responders resorted to manual interpretation of traffic photos, social media posts, and other indirect sources to take their best guesses at whether their travel routes were safe, researchers found. This approach not only provided imperfect information but also increased response times, resulting directly in more severe storm impacts.
The researchers used Houston as a case study, inputting historical hydrological and physical data from Hurricane Harvey into their framework to determine how much of the city’s roadways the AI program could assess based on available information at the time.
“The model was able to observe around 37,000 road links, which is around 60% of the network that we considered, and that is a significant improvement,” Panakkal said.
While researchers acknowledge that further development is necessary to improve the model’s predictive capabilities, they stress that this proof of concept demonstrates a workable solution that may one day assist emergency decision-making in cities around the world.
“Considering climate change impacts and climate-exacerbated weather events, the frequency and intensity of flood events could increase in the future, so we need a solution to better respond to flood events and their impacts on infrastructure,” Padgett said.
Access the study, “More Eyes on The Road: Sensing Flooded Roads by Fusing Real-Time Observations From Public Data Sources,” in Reliability Engineering & System Safety.
Top image courtesy of Hans/Pixabay
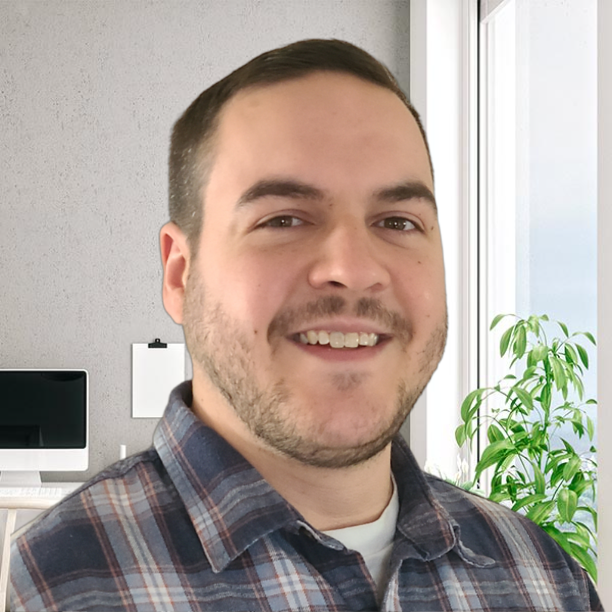
ABOUT THE AUTHOR
Justin Jacques is editor of Stormwater Report and a staff member of the Water Environment Federation (WEF). In addition to writing for WEF’s online publications, he also contributes to Water Environment & Technology magazine. Contact him at jjacques@wef.org.