Conventional approaches to studying rainfall patterns across both large distances and long timeframes suffer from a Goldilocks problem. For example, while a rain gauge may provide comprehensive precipitation data for a specific point on a near-constant basis, accurately capturing the variable nature of rainfall across a watershed may require researchers to deploy too many gauges to be practical. On the other hand, satellites and other large-area measurement methods often read rainfall at a specific point too infrequently to produce much useful data for municipalities, farmers, and meteorologists.
According to new research from Germany’s Karlsruhe Institute of Technology (KIT) and University of Augsburg (UA), infrastructure that already exists in nearly every corner of the world may offer a solution that is just right.
Mobile-phone networks often rely on towers equipped with commercial microwave link (CML) antennas, which communicate via radio signals to coordinate calls across long distances. Rainstorms weaken these signals, and according to the research team, analyzing fluctuations in CML signal strength can translate into accurate rainfall measurements across both space and time.
“We have developed a completely new method for rain measurement from this interaction between weather events and human technology,” said KIT climatologist Harald Kunstmann in a release about the new research. “If a CML network is in place, we neither need new infrastructure nor additional ground staff.”
Stronger Readings From Weaker Signals
Partnering with a large, Swedish telecommunications company, the research team studied the radio frequencies emitted by nearly 4,000 CML antennas on a minute-by-minute basis for a year. The monitored CML network, which stretches across Germany, represents a diverse range of climate zones from the North German Plain to the Alps.
The measurement process begins by focusing on instances in which CML frequencies weaken to wavelengths between 15 to 40 GHz, which the researchers describe corresponds to the typical size of the raindrops that disrupt radio waves. By considering differences in signal strength alongside the location of specific CML antennas, the researchers developed Germany-wide precipitation maps estimating rainfall occurrence and volume as conditions changed.
However, simply monitoring for a specific wavelength did not provide sufficient information to infer rainfall accurately. Other factors, such as high winds, dew on CML antennas, or the refraction and reflection of ultraviolet light, can also weaken CML signals to the target range. Because eliminating the influence of these phenomena manually would take an enormous amount of time for a dataset of this size, the research team turned to artificial intelligence (AI).
A convolutional neural network used by the team has the computing power to consider up to 140,000 parameters independently when analyzing data. Comparing readings from CML signals during the study period alongside actual, hourly measurements conducted by the German Meteorological Service (GMS) using conventional means “taught” the AI to identify the degree to which weaker CML signals were caused by rainfall rather than other factors.
“With the help of our AI, we were able to identify the signal attenuation that was due to rainfall,” described Julius Polz, KIT doctoral student, in the release. “We have now trained our AI in such a way that we no longer need to calibrate the system using traditional methods of rain measurement.”
Accuracy With Limitations
After a lengthy learning process, the team’s AI demonstrated impressive accuracy in measuring rainfall across both space and time. During spring and summer of 2018, for example, the AI successfully identified 76% of all rain events documented by GMS and more than 90% of events that delivered more than 0.6 mm (0.02 in.) of rain per hour. Compared to manual methods of distinguishing between the effects of rainfall and those of other sources, the AI reduced false rainfall detections by up to 51%, the researchers report.
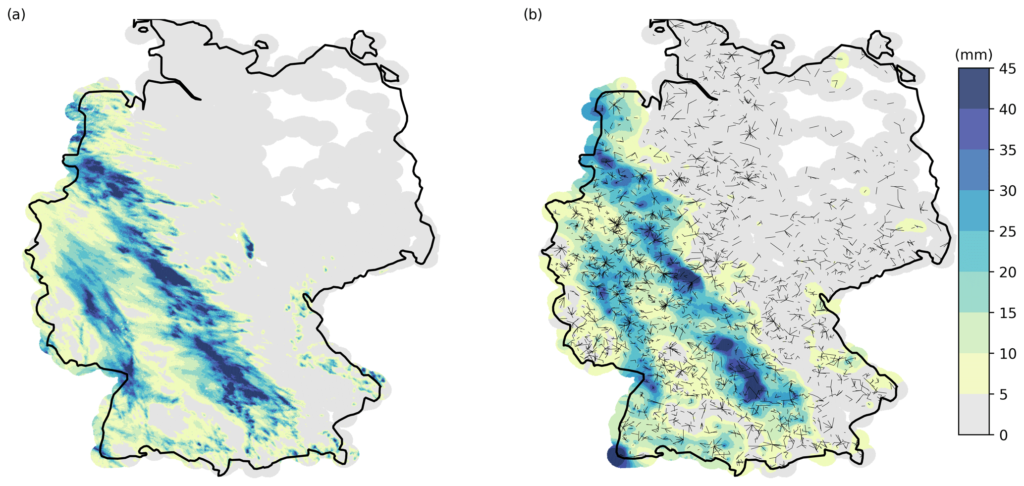
Study authors are confident that the trained AI can now develop accurate rainfall maps in almost any location with an existing CML network, even in areas without available reference data for rainfall occurrence. However, the researchers note that the method is not suitable for all climates or all seasons. Sleet and freezing rain typically cause much larger disturbances to CML signal strength than rainfall alone, and tests show that the effects of melting snow on CML antennas can result in rainfall readings as much as four times higher than actual figures.
For the AI’s next field test, the researchers plan to measure long-term rainfall patterns across Burkina Faso in West Africa, describes the KIT release. While Burkina Faso lacks comprehensive data about rainfall, which could be of immense value to the country’s farmers, it maintains a consistently warm climate and an array of towers equipped with CML technology.
Read more about the team’s research into using mobile-service towers to measure rainfall in the journals Hydrology and Earth System Sciences and Atmospheric Measurement Techniques.