Global climate models that help researchers predict precipitation timing, location, and volume suffer from a weakness popularly known as “the drizzle problem”.
In simple terms, the drizzle problem refers to the tendency of large-scale climate models to overlook smaller-scale atmospheric factors that cause routine storms to deliver less or more precipitation than average. These models more often underestimate precipitation intensity. For instance, they might anticipate light drizzles instead of actual torrential downpours. This reduces their usefulness, especially as extreme precipitation occurs ever more frequently with climate change.
The solution to the drizzle problem, contends new research from Columbia University (New York) climatologists, relies on accounting for how clouds move and interact with one another to affect rainfall. However, this is difficult for two reasons. For one, the interplay between cloud organization and precipitation during a storm occurs on scales too small for most climate models to capture. For another, it requires something that has not existed until now: a quantitative measure that can accurately represent cloud organization.
A recent study published in the Proceedings of the National Academy of Sciences describes how a combination of machine learning and artificial intelligence can help solve the drizzle problem by addressing both issues.
“Our findings are especially exciting because, for many years, the scientific community has debated whether to include cloud organization in climate models,” Pierre Gentine, study co-author and Director of Columbia University’s LEAP Center, said in a statement. “Our work provides an answer to the debate and a novel solution for including organization, showing that including this information can significantly improve our prediction of precipitation intensity and variability.”
Dissecting the ‘Drizzle Problem’
Cloud organization is an important variable to consider when attempting to understand how the same storm might deliver more precipitation to one area than another. When clouds cluster together, the surrounding atmosphere becomes more humid, which generally correlates with higher rainfall volumes within a narrow water column. These movements are poorly captured by global climate models, which typically have a resolution no finer than 100 km (62 mi).
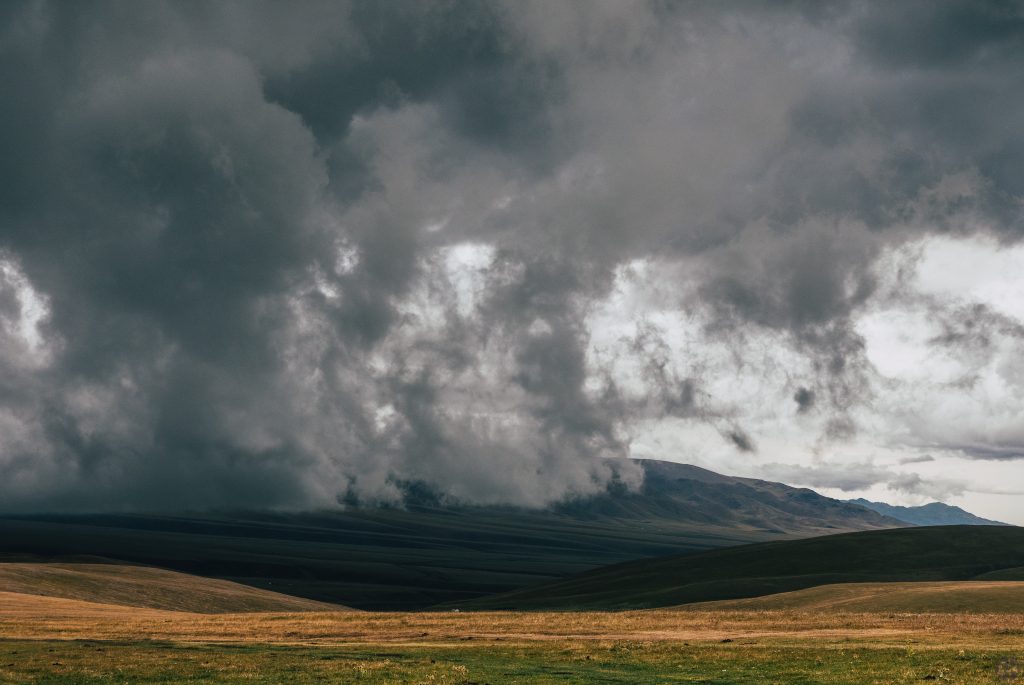
To compensate for clouds and other phenomena that occur at smaller scales, climate models rely on a technique called stochastic parameterization — using historical data as well as other observable factors to infer such conditions as cloud organization based on probability. However, since light drizzles tend to occur far more often than heavy deluges in most of the world, parameterization by climate models often assumes deviations from normal rainfall will be sprinkles rather than inundations.
The proper metric to quantify cloud organization could eliminate the need for parameterization and make predictions by climate models more actionable at local scales. During the past few decades, at least 20 such metrics have been proposed in scientific literature, according to the study. However, these existing metrics were designed to either measure cloud movement and organization over areas too expansive to be actionable for predicting rainfall or sought to represent only specific aspects of cloud behavior that are irrelevant to precipitation.
Mimicking the Human Brain
Existing metrics for cloud organization were developed according to certain assumptions or foundations. For example, they might describe cloud clustering as a function of other climatological variables, or adapt existing formulas and measures originally intended to characterize other phenomena. In fundamental contrast to existing metrics, the Columbia study describes tasking an advanced artificial intelligence with developing an optimal way to measure cloud behavior based solely on the patterns it observes elsewhere in the climate model.
Researchers began this process by training a neural network algorithm — an artificial intelligence designed to recognize patterns in a manner that mimics the human brain — on data from a high-resolution series of simulated storm clouds provided by a publicly available climate model. The neural network delved into the patterns between when clouds began to form and when the accompanying storm dissipated. By studying the network’s observations of these patterns, researchers managed to interpret and isolate the numbers the algorithm assigned to cloud organization.
When the researchers modified global climate models to use the new metric, the models managed to predict extreme rainfall events with roughly double their usual accuracy, study results illustrate. This new metric not only offers the potential to improve how large-scale climate models predict precipitation, but also several other small-scale factors currently missed by these models, according to the researchers.
“We discovered that our organization metric explains precipitation variability almost entirely and could replace a stochastic parameterization in climate models,” said Sarah Shamekh, Columbia Ph.D. student and lead study author, in a statement. “Including this information significantly improved precipitation prediction at the scale relevant to climate models, accurately predicting precipitation extremes and spatial variability.”
Read the full study, “Implicit Learning of Convective Organization Explains Precipitation Stochasticity,” in the Proceedings of the National Academy of Sciences.
Top image courtesy of U.S. Department of Energy
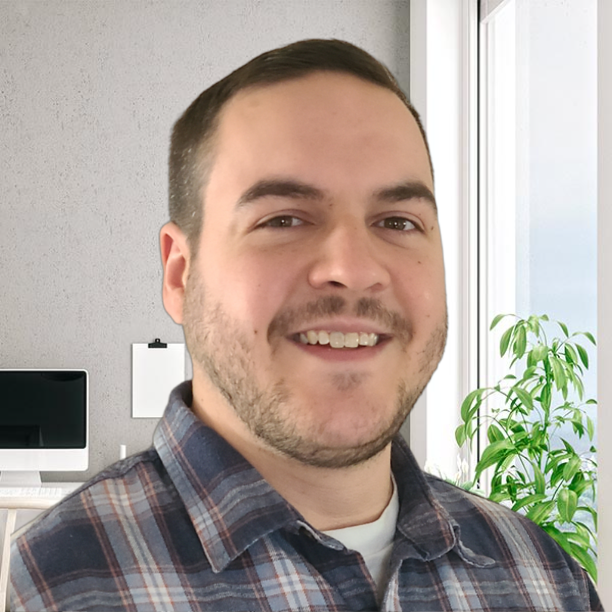
ABOUT THE AUTHOR
Justin Jacques is editor of Stormwater Report and a staff member of the Water Environment Federation (WEF). In addition to writing for WEF’s online publications, he also contributes to Water Environment & Technology magazine. Contact him at jjacques@wef.org.