Predicting the size and expected runoff volumes from hailstorms is notoriously difficult, according to researchers with the U.S. National Center for Atmospheric Research (NCAR). Because cloud systems that produce rain often have different features than those that produce hail, conventional prediction methods often fail to yield accurate forecasts.
NCAR researchers investigating the challenge of hailstorm prediction recently published a study in the American Meteorological Society’s Monthly Weather Review journal that details how a technology typically used in security systems and smartphones might make the job of predicting hailstorms easier.
Pattern-processing predicts hailstorms
As fallen ice melts, hailstorms produce runoff much in the same way as other forms of precipitation. In addition to conveying ground-level pollutants into waterways, large-diameter hail can also cause damage to property and agriculture.
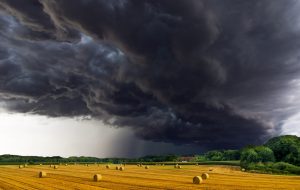
Because the measurable conditions that dictate whether a storm will produce a certain type of precipitation differ, conventional methods to predict the severity of rainstorms are not as effective when predicting hailstorms. A new study from the U.S. National Center for Atmospheric Research (NCAR) details how researchers are adapting the machine-learning programs behind facial recognition systems to better predict the occurrence and severity of hailstorms. Photo courtesy of pixel2013/Pixabay
The first step in predicting the severity of a hailstorm is determining whether to expect hail or another form of precipitation based on gathering clouds and atmospheric conditions, described NCAR scientist David John Gagne, who led the recent research.
“We know that the structure of a storm affects whether the storm can produce hail,” Gagne said in a release. “A supercell is more likely to produce hail than a squall line, for example. But most hail forecasting methods just look at a small slice of the storm and can’t distinguish the broader form and structure.”
NCAR scientists employed the same artificial intelligence (AI) technique as the one that drives facial recognition systems – known as a convolutional neural network — to examine such factors as air humidity, cloud temperatures, and wind speed instead of eyes, noses, and mouths.
Trained on data from just 30 days in spring of 2016, NCAR’s AI can predict whether to expect hail as well as the chances that hail size will exceed 25 mm (1 in.) over the hour following storm detection. Through a form of deep learning, the NCAR network taught itself how to synthesize information about the environment and storm morphology, revealing a deep understanding of hail growth and new information about how hailstorms form differently than rainstorms, according to the researchers.
Whereas conventional computer models struggle to quantify the vast array of factors that might influence a storm’s severity and instead draw conclusions from small samples, the convolutional neural network pores over large amounts of data and looks for pre-determined shape patterns based on storms that have produced hail in the past.
“The shape of the storm is really important,” Gagne said. “In the past we have tended to focus on single points in a storm or vertical profiles, but the horizontal structure is also really important.”
Training and improving the network
Gagne and his team trained the convolutional neural network in much the same way as computer engineers train facial-recognition systems: through images. After simulating several hailstorms using NCAR’s Weather Research and Forecasting model, the researchers had their AI system analyze snapshots of the visible conditions leading up to the storm. Combined with historical data about the relationship between these conditions and resulting hail size, the network taught itself to recognize patterns in a way that “statistically significantly outperforms all other methods,” reads the study.
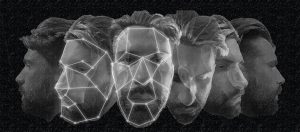
Computer engineers train facial recognition systems to recognize such features as eyes, noses, and mouths using a large number of facial images. The NCAR team trained their hail-predicting artificial intelligence in a similar way, combining quantitative data with images of simulated hailstorms to teach the system the patterns it should search for to accurately predict hailstorm occurrence and severity. Photo courtesy of N. Hanacek/U.S. National Institute of Standards and Technology
The process of training and testing the AI network confirmed storm features already identified by NCAR to produce hail, Gagne said. By analyzing historical patterns, the network identified that storms with lower-than-average pressure near the surface and higher-than-average pressure near the storm top are more likely to produce severe hail. Storms with a “circular” shape that revolve around a low-pressure center are also likely hailstorm candidates, the network found.
The NCAR team is now working to improve the network’s accuracy by training it on images of real-world storms and radar-estimated hail. With an eye toward the technology’s operational use, Gagne and his team are collaborating with researchers from the University of Oklahoma (Norman) to enhance the network’s predictive capabilities.
“I think this new method has a lot of promise to help forecasters better predict a weather phenomenon capable of causing severe damage,” Gagne said. “We are excited to continue testing and refining the model with observations of real storms.”
Read the NCAR team’s study, “Interpretable Deep Learning for Spatial Analysis of Severe Hailstorms,” in Monthly Weather Review.